Machine Learning in Healthcare: 5 Use Cases that Improve Patient Outcomes
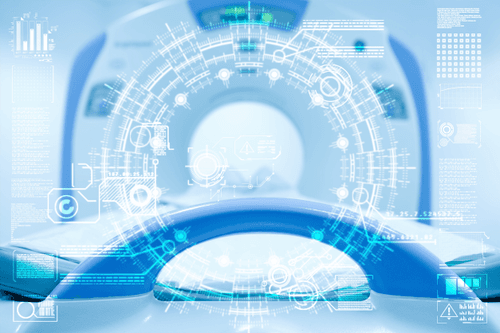
Team Anaconda
5min
Artificial intelligence (AI) is all around us: It’s in our intelligent home devices, healthcare diagnostic tools that scan our bodies, and cars we drive or pass on the street. AI has revolutionized the way we live, and it’s doing the same where we work. Increasingly, organizations are adopting AI and using AI platforms and other technologies to develop, deploy, and maintain AI-powered products and services at enterprise scale.
According to a McKinsey survey (The State of AI in 2022), the rate of enterprise AI adoption has more than doubled since 2017, and organizations that generate the highest financial returns from AI pull ahead of their competitors. McKinsey survey respondents cited use cases spanning a range of activities, with 24% applying AI for service operations optimization, 20% applying AI to create new AI-based products, and 19% applying AI for customer service analytics, customer segmentation, and new AI-based product enhancements.
However, to capture the business value of such AI use cases, organizations must navigate a dizzying array of tools and complex techniques. That’s why many enterprise organizations are creating bespoke AI platforms using a combination of trusted providers and open-source technology. This approach allows them to leverage both the reliability and support of an enterprise AI platform and the innovation and community of open-source tools.
Let’s take a closer look at the kinds of AI that enterprise organizations are building with these tools.
McKinsey’s research shows that organizations have doubled the average number of ways they use AI, up from 1.9 capabilities in 2018 to 3.8 in 2022. Among these, robotic process automation, computer vision, and natural language processing remain the most commonly deployed technologies, followed by chatbots and deep learning.
Based on Anaconda’s decade of working with enterprise organizations applying AI to innovate products and services and optimize operations, here are 10 common AI use cases for the enterprise that we’ve observed:
1. Task automation: AI can automate manual tasks, such as data entry, customer service follow-up, and product recommendations. This can free up employees to do other tasks that can improve efficiency and save money. Automation can be used to search for and identify sales leads or surface new strategies for marketing departments, based on the success of past content and campaigns.
2. Customer service improvements: AI can be used to provide better customer service, using automated tools like chatbots and virtual assistants. AI can search customer emails and support recordings for patterns in complaints that can inform product and service improvements. AI can automate tasks that assist customers around the clock to provide a more personalized experience and resolve issues faster.
3. Fraud detection: The banking industry uses AI detection algorithms to detect fraud in a matter of seconds. These models use data such as cardholder identification, the location where the card was issued, the time the transaction took place, the transaction location, and the transaction amount to identify fraud. Once the model detects an anomaly, a notification system can be programmed to block the transaction and alert fraud detection services the moment the model identifies a suspicious transaction. Fraud-detection algorithms can be applied in many other areas, such as network intrusion detection.
4. Data analysis to uncover insights: Using data, machine learning, and natural language processing, AI can help enterprise organizations make sense of their data faster, by surfacing insights and patterns to analyze. AI can be used to gauge sentiment in text and predict an individual’s intentions using their social media comments, reviews, surveys, phone scripts, or customer service chats. These insights allow for quicker and informed decision making that can uncover opportunities to improve existing products, flag issues before they affect a large number of customers, create new products or services, drive marketing campaigns, and improve operations. These are just a few of the ways these insights can be used.
5. Security protection: AI makes it possible to detect security threats in real time through the use of things like authentication methods for password protection, the use of CAPTCHA to detect human versus bot, facial recognition, and fingerprint scanners. These solutions, made possible with machine learning, can help prevent cyberattacks, identify bad actors online, and strengthen an organization’s defenses to safeguard their data, customers, and employees from threats.
6. Predictive maintenance: Using predictive analytics, AI can be used to predict when equipment is likely to break down so repairs can be carried out before problems occur. Highly accurate anomaly detection algorithms can detect issues down to a fraction of a millimeter and flag them for human operators so they can intervene and prevent failures of critical equipment and safety systems. This can prevent accidents, improve equipment uptime, and reduce maintenance costs. Reliable predictive maintenance systems help ensure customer safety, satisfaction, and retention.
7. Supply chain optimization: Throughout the supply chain, analytical models are used to identify demand levels for different marketing strategies, sale prices, locations, and many other data points. Predictive analysis dictates the inventory levels needed at different facilities. Optimization models help guide the exact flow of inventory from manufacturer to distribution centers and ultimately to customer-facing storefronts. Machine learning is helping parts and vehicle manufacturers—and their logistics partners—be more efficient and profitable, while enhancing customer service and brand reputation.
8. Intelligent search: AI can be used to power intelligent search engines, which can understand user queries and find the most relevant results faster than traditional search engines. This can improve the usability of search engines and make it easier for users to find what they’re looking for online. A great example of this in action is OpenAI’s ChatGPT, a chatbot that is built on the language models of GPT-3 and trained with deep learning techniques that enable it to perform with such speed that some have predicted ChatGPT poses a threat to Google’s search engine.
9. Patient diagnosis: Visual data processing for medical image analysis is used in healthcare to assist radiologists in reading medical images faster for diagnosis and disease detection. Researchers have developed deep learning algorithms trained on previously captured radiographic images to recognize the early development of tumors in the lungs, breasts, brain, and other parts of the body. Algorithms can be trained to recognize complex patterns in radiographic imaging data and provide diagnostic information 30 times faster than a human can, contributing to faster and more accurate diagnoses.
10. Product recommendations: Ecommerce companies use AI to analyze customers’ purchases and pageviews so they can recommend more relevant products to enhance targeting and reach the right audiences with more successful ad campaigns. Predictive analytics is also used in anticipatory shipping models, predicting customer product patterns and ensuring preferred items are stocked in the nearest warehouses. AI is also used for price optimization, allowing companies to provide discounts on popular items and earn profits on less popular items.
If you want to develop AI applications, Anaconda makes it easy to get started. It’s simple to set up and provides access to a wide range of open-source data science and machine learning tools, frameworks, and libraries. Simply download and install it on your system or access Anaconda’s practitioner tools, like cloud-hosted notebooks.
Anaconda is powered by the open-source community, giving you access to a community of more than 30 million tool builders and maintainers, model makers, and user-practitioners. Enterprise teams can leverage Anaconda’s integration, data security, and administrative tools to empower users and teams to collaborate more efficiently and achieve business value faster.
To learn more about how enterprise data science and machine learning practitioners are using Anaconda to build AI faster and more collaboratively across their organizations, contact us today.
Talk to one of our experts to find solutions for your AI journey.