Why Power Efficiency Is Key to AI Innovation, and What It Means for Hardware and Software Makers
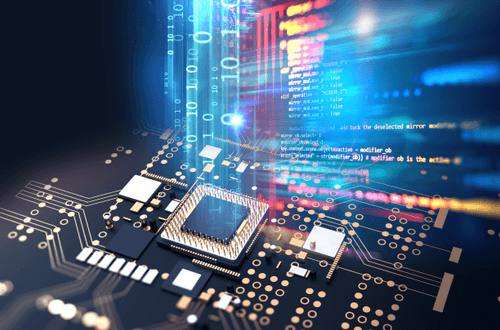
Team Anaconda
4min
Machine learning is accelerating the pace of scientific discovery across fields, and medicine is no exception. From language processing tools that accelerate research to predictive algorithms that alert medical staff of an impending heart attack, machine learning complements human insight and practice across medical disciplines.
However, with all the “solutionism” around AI and machine learning technologies, healthcare providers are understandably cautious about how it will really help patients and bring a return on investment. Many AI solutions on the market for healthcare purposes are tailored to solve a very specific problem, such as identifying the risk of developing sepsis or diagnosing breast cancer. These out-of-the-box AI solutions make it difficult or impossible for companies to customize their models and get the most out of their investment.
Open-source data science allows healthcare firms to adapt models to address a variety of challenges using the latest machine learning technologies, such as audio and visual data processing. Using open-source tools, data scientists can custom-build applications in a way that meets healthcare IT’s strict requirements and improves patient care in a variety of settings, ultimately differentiating an organization from its competitors. Here are five machine learning use cases for the healthcare sector that can be developed with open-source data science tools and adapted for different functions.
A study conducted by the New England Journal of Medicine last year found 83% of respondents reported physician burnout as a problem in their organization. Half of them reported that “off-loading administrative tasks” would help remedy the problem, allowing physicians to spend more time with patients. A significant portion of these administrative tasks involve reviewing and updating Electronic Health Records (EHRs). Nearly every hospital in the U.S. uses an EHR system and so do most clinics. Improving the efficiency of updating EHRs is a high priority for most. This is where NLP tools come in.
By leveraging NLP tools that use algorithms to identify and categorize words and phrases, physicians can dictate notes directly to EHRs during patient visits. Doctors and patients alike can review charts and summaries neatly compiled by NLP tools instead of having to read through notes and test results to understand a patient’s overall health. By spending less time maintaining EHRs, physicians can spend more time with their patients.
Around the world, healthcare providers have begun using tools built from machine learning models that use anomaly detection algorithms to predict heart attacks, strokes, sepsis and other serious complications. These tools use data from patients’ historical records, daily evaluations, and measurements of vital signs in real-time, such as heart rate and blood pressure, to alert staff of imminent patient risks so they can immediately take preventive actions.
One example is El Camino Hospital. Their researchers used electronic health records, bed alarm data, and nurse call data to develop a tool for predicting patient falls. This new tool alerts staff when a patient is at high risk for falling so they can take action to reduce the risk. They managed to reduce falls by 39%. According to the Joint Commission for Transforming Healthcare, an in-patient injury due to a fall adds an average of 6.3 days to a hospital stay and costs $14,000. Another example is the Sepsis Sniffer Algorithm (SSA) developed by the Mayo Clinic. The SSA uses demographic data and vital sign measurements to trigger an alert whenever the risk of developing sepsis increases, cutting manual screening time by 72%. This allows doctors and nurses to spend more time treating the illnesses patients came to them for in the first place.
Scientists and physicians would have to read and process an overwhelming quantity of reports and studies to keep up with trends in specific areas of medical research. For example, academics published more than 342,000 articles on drug evaluation and analysis alone between 2007 and 2016. Using NLP tools and neural networks to parse literature will provide medical researchers with valuable insights in the years ahead.
For example, a team of researchers from the U.S. and Ireland worked together to conduct a study on Adverse Drug Events (ADEs) using text mining, predictive analytics, and neural networks to analyze vast databases of medical literature and social media posts for comments related to drug side effects. After analyzing over 300,000 articles from medical journals and over 1.6 million comments on social media, the team used data visualization tools to show the relationships between drugs and side effects.
NLP is also being used to mine unstructured data in EHRs for insights, such as data from electrocardiogram results or copies of manually written notes that were uploaded to a patient’s record, but not entered into form fields. cTAKES is one example of an open-source NLP project by Mayo Clinic, Boston Children’s Hospital, and other organizations to develop a tool that parses unstructured data in EHRs to extract insights.
Radiologists’ workloads have increased significantly in recent years. Some studies found that the average radiologist must interpret an image every 3-4 seconds to meet demand.Researchers have developed deep learning algorithms trained on previously captured radiographic images to recognize the early development of tumors in the lungs, breasts, brain, and other areas. Algorithms can be trained to recognize complex patterns in radiographic imaging data. They can detect breast cancer from mammograms with remarkable accuracy. One early breast cancer detection tool developed by the Houston Methodist Research Institute interprets mammograms with 99% accuracy and provides diagnostic information 30 times faster than a human. Tools like these also decrease the need for biopsies. Most radiologists agree that these tools help them to improve patient care. They make them better at their jobs, but do not replace them.
CNNs are powerful tools for recognizing and classifying images. Several researchers have used them to develop machine learning models for skin cancer detection with 87-95% accuracy using TensorFlow, scikit-learn, keras and other open-source tools. In comparison, dermatologists have 65% to 85% accuracy rate in detecting melanomas. Models are trained using thousands of images of malignant and benign skin lesions. One example of an open-source project like this one is available to the public on Github. In addition to skin cancer diagnosis, researchers are also using CNNs to develop tools for diagnosing tuberculosis, heart disease, Alzheimer’s Disease and other illnesses.
While healthcare organizations must be more prudent than most other industries about security, governance, and compliance, they can still train machine learning models using anonymized data to comply with HIPAA requirements. Ensuring the integrity of your software environment is crucial for handling real user medical data. Anaconda Enterprise, provides a stable and secure environment for practitioners in highly regulated fields to work with breakthrough open-source machine learning technologies. It also provides access to a secure, governable package repository so data scientists can access IT-approved data science packages in the development of innovative models.
Talk to one of our experts to find solutions for your AI journey.