Behind the Code of Dask and pandas: Q&A with Tom Augspurger
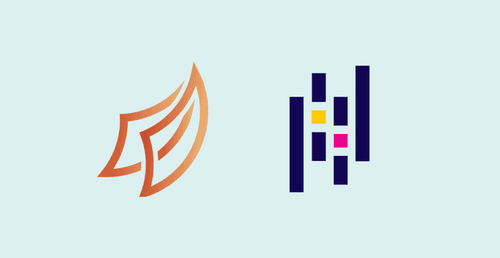
Peter Wang
5min
By: Peter Wang & Natalie Parra-Novosad
As researchers, journalists, and many others have discovered, machine learning algorithms can deliver biased results. One notorious example is ProPublica’s discovery of bias in a software called COMPAS used by the U.S. court systems to predict an offender’s likelihood of re-offending. ProPublica’s investigators discovered the software’s algorithm was telling the court system that first-time Black offenders had a higher likelihood of being repeat offenders than white offenders who had committed multiple crimes. They also found only 20% of the individuals predicted to commit a violent crime did so. Discoveries like these are why ethical AI is top-of-mind in Silicon Valley and for companies around the world focused on AI solutions.
While it’s true machine learning has had problems with biased outcomes, one thing is for certain: humans are the reason for that. AI mirrors our biases, and it is our best chance at examining subconscious bias. Why? AI is not inherently biased. It doesn’t care whether it’s right or wrong. It doesn’t care how exciting or new an idea is (novelty bias). It doesn’t have feelings and emotions tied to past experiences. It makes purely logical decisions based on the data it has been provided. Researchers have found that machine learning can improve human decision-making and reduce racial disparity when thoughtfully developed and implemented.
Because AI is already being used to make life-changing decisions, it is crucial to minimize bias and maximize fairness. Many companies have proceeded with the development of ML models and AI applications before establishing organization-wide values and ethical standards. As we have seen, this is putting the cart before the horse. The following are a few ways organizations should proactively address bias in AI technologies.
A lack of diversity in staff and diversity in data has led to several recent problems with AI solutions, such as the inability of facial recognition algorithms to correctly identify those with darker skin. The key to preventing these kinds of problems in new technologies is diversity – diversity in staff and diversity in data, which leads to diversity in the questions asked and the options explored. Researchers at the AI Now Institute reported that only 12% of researchers who contributed to the three leading machine learning conferences in 2017 were women and only 10-15% of AI researchers at major tech companies are women. They found there is no reliable data on racial diversity, and limited evidence suggests the percentage of women in AI is even lower than those in computer science in general. It is clear the field of AI and machine learning needs to become more diverse to create models that produce predictions that more accurately reflect our values.
A major part of the difficulty in ensuring AI makes unbiased decisions lies in the definitions of fairness. What does fair mean when it comes to machine learning? Machine learning scholars, as well as scholars in the social sciences, have begun to debate this question. There are multiple definitions of fairness, and it’s impossible for one machine learning algorithm to fulfill all of them.
Here are a few different types of fairness to consider:
Some people think that fairness might be achieved in machine learning (ML) with blindness, which means removing protected attributes from data sets, such as gender and race. However, there are usually other attributes, like neighborhood or zip code, that can become proxies for protected attributes. Second, in some cases, it may be unfair and ineffective to remove protected attributes, like in the case of machine learning algorithms that make medical diagnoses.
Data scientists can calculate trade-offs between different types of fairness and optimize models, but ultimately fairness is not a number. We have to explore moral, structural, historical, and political contexts of data and ML outcomes. This is where ethicists come in.
According to KPMG, ethicists are among the top 5 AI hires a company needs to succeed in 2019. In Deloitte’s 2018 State of AI in the Enterprise, they reported one-third of executives cited ethical risks as a top concern. While people developing AI solutions should be thinking about their impact from an ethical standpoint, this can often be difficult with goals of efficiency and business value that are often their raison d’etre within the organization. Some companies rely on a legal team to help ensure their products or solutions are meeting legislative requirements, but this is not the same as thinking about an AI solution from an ethical standpoint. Whether a solution is legal or not, an unethical product can be damaging enough to provoke new regulations and severely injure a company’s reputation.
Form an ethics committee that includes people from diverse backgrounds from a variety of departments in the company (not just IT and data science). Also include ethicists and legal staff on this committee. With fairness, diversity, and bias in mind, formulate standards that all staff are to follow everyday in the development and promotion of new AI technologies.
According to an article on tackling bias in AI from McKinsey, “Underlying data are often the source of bias.” We agree with this statement 100%. One reason for this is that the criteria humans have used to make decisions in the past is unfair. For example, if a machine learning model is tasked with predicting the best candidate for a nursing job, and the pool of data the model was trained on contains very few men, the algorithm will likely not indicate that a man is the best person for the job, regardless of other qualifications. The same would be true of a model that was trained to find the best CEO using a data set that contained mostly male candidates. Once this model goes into production, it will recommend men as the best candidates for CEOs. We recently saw this bias play out in an algorithm used to serve ads for job openings. Ads for higher-paying jobs were shown more frequently to men.
An AI system itself cannot determine if bias has been sufficiently minimized. Algorithmic systems must be developed to support human values like justice and fairness. Humans must determine if AI meets our legal and ethical standards, and organizations should start by determining what their ethical standards are when it comes to ML and AI. In the future, AI could guide fairer human decision-making. Diverse groups of humans could compare their decisions to those made by AI, improving decision-making at the organizational and societal levels.
Talk to one of our experts to find solutions for your AI journey.