Anaconda’s Q1 2023 Open-Source Roundup
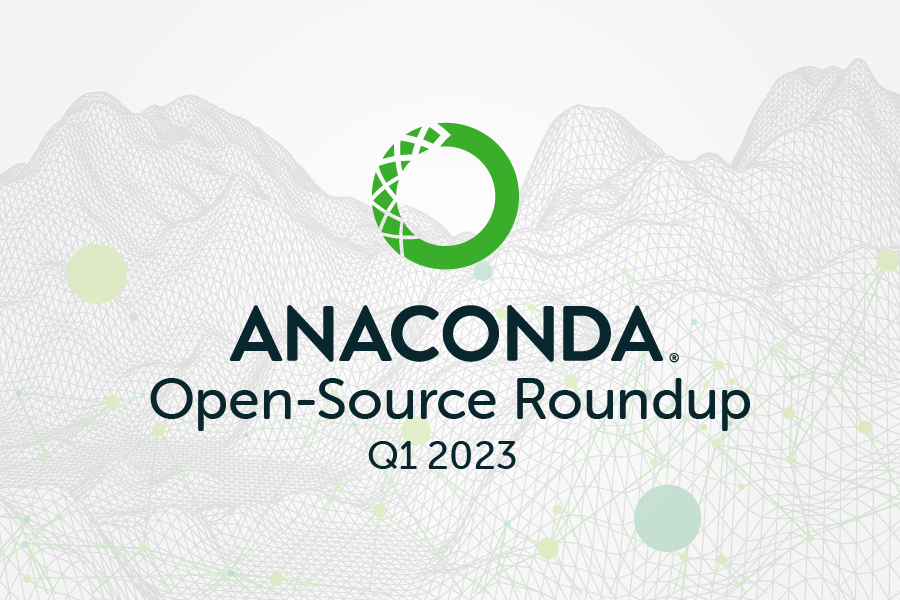
Joseph Currenti
3min
AI technology has come a long way in recent years, and it’s become an invaluable tool for businesses looking to stay ahead of the competition. But there’s no such thing as a one-size-fits-all AI platform. Every organization has its own objectives and requirements and its own deployment strategies, so it’s important to select an AI platform that meets your needs.
In research summarized in the Data and AI Leadership Executive Survey 2022, 92% of companies reported strong returns on their data and AI investments, up from 48% in 2017 and 70% in 2020. The vast majority (96%) reported their organizations were in a pilot or AI rollout with limited production.
An AI platform is a software solution that enables organizations to develop, deploy, and manage AI applications at scale. It should provide a complete set of capabilities for data science and machine learning teams to build, train, and deploy models into production.
An enterprise AI platform must unify AI models, data processing, orchestration, storage, workflow, integration, and monitoring with an integrated solution that supports building applications and ingesting AI into the current technology stack. Luckily, there are options and opportunities for organizations of all sizes to explore.
Here, we will explore five capabilities your AI platform should have to support your teams in fully realizing AI’s potential. From natural language processing (NLP) to machine learning (ML) and beyond, these capabilities are essential for any company looking to get the most out of their AI investments.
As your business grows, so does the need for scale, agility, and automation. The right AI platform will give you the ability to quickly develop and deploy models that drive real-time decisions and improve customer experiences.
Here are 5 capabilities your AI platform should have:
1. Automation: A great AI platform will help your team accelerate the automation of proven models with consistently solid performance by applying automation strategically. Your machine learning and data science environments should be easy to integrate with the latest and best tools for your use cases.
2. IT enablement and governance: Maintaining control over the software supply chain is key to harnessing the power of AI. While users must be able to share and collaborate on projects easily, IT administrators must be able to provision and deactivate user accounts and have visibility to where and how business and customer data is being used so they can identify and address issues and track details that will be important for audits.
3. Scale: Machine learning practitioners must be able to scale model training, which means they need centralized workflows that support collaboration with others. In the age of GPUs and TPUs, this level of scale in model training has become economically feasible. As a result, today’s challenge is not finding the computing power, but managing an environment that supports it.
Thus, deploying massive amounts of compute requires significant supporting infrastructure. Your AI platform should be able to handle your organization’s requirements, use cases, and throughput as you scale your AI application.
4. Security: It is paramount for chief security information officers (CISOs) and IT administrators to secure the open-source software supply chain. Open-source packages are commonly used by data scientists to develop and test machine learning models, assessing their accuracy, scalability, and a multitude of other factors. As part of this process, data scientists often download software packages directly to their laptops.
Public sources come with additional risks that must constantly be monitored and mitigated, putting IT administrators at a disadvantage. If you are planning to deploy machine learning models into production, you should choose an AI platform with maintainers and authors who are familiar with the tools and techniques your team will use. By incorporating bugs and feedback from the open-source community, great AI platforms will be able to monitor vulnerabilities in open-source packages and tools through automation and manual curation.
5. Support: A critical component of any AI platform is support, which is often one of the last capabilities enterprise buyers look for. Teams need reliable bug reporting and tracking, engaging training resources, and ongoing support when working with open-source software. Look for an AI platform that comes backed by a team of experts who understand the challenges of developing AI and are capable of providing enterprise support at scale. Ask how they prioritize support when your users are affected and your team does not have a workaround option.
Check out the list of questions we developed to ask your AI platform provider in The Definitive Guide to AI Platforms for Open-Source Data Science and ML.
Anaconda is built for the enterprise and powered by the open-source community, giving you access to collaboration resulting from more than 35 million tool builders and maintainers, model makers, and user-practitioners.
With Anaconda, enterprise teams get access to the best tools and repositories for their use cases, and security teams get the data security and administrative tools to empower users and secure the software supply chain so everyone can collaborate more efficiently and achieve business value faster.
To learn more about how enterprise data science and machine learning practitioners are using Anaconda to build AI faster and more collaboratively across their organizations, contact us for a demonstration today.
Talk to one of our experts to find solutions for your AI journey.