A Comprehensive List of Python in Excel Resources
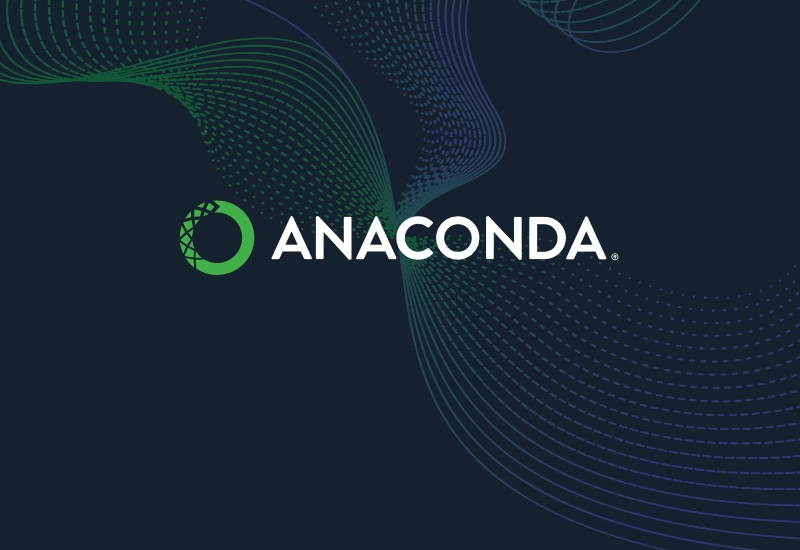
Daniel Rodriguez
For decades, AI has relied on real-world data as its backbone, fueling everything from predictive text to autonomous vehicles. However, as the scale and complexity of AI systems have exploded, so too have the challenges in acquiring, curating, and safeguarding real-world data. Enter synthetic data—a transformative approach to dataset generation that addresses these challenges and opens entirely new frontiers for AI development.
Synthetic data is artificially generated data that mirrors the properties of real-world datasets. Unlike traditional data, it can be tailored to specific needs, created in infinite quantities, and designed with built-in safeguards to respect privacy and fairness. While it may seem like a niche solution, synthetic data is rapidly becoming indispensable in the development and evaluation of cutting-edge AI models.
One of the most compelling examples comes from the automotive industry. Companies like Waymo and Tesla rely on real-world data gathered from an array of sensors covering the vehicle plus synthetic data to simulate millions of driving scenarios that would be impractical, dangerous, or impossible to capture in the real world. From testing how an autonomous vehicle might react to a pedestrian jaywalking in heavy fog to simulating rare traffic conditions, synthetic data has become a cornerstone of the industry’s rapid advancements.
Similarly, the healthcare sector is experiencing a synthetic data renaissance. Startups and research institutions are using generative models to create synthetic medical records, enabling the training of AI systems without compromising patient privacy. For example, synthetic CT scans and MRI data are helping train diagnostic algorithms to identify rare diseases, often with greater accuracy than real-world datasets due to their balanced representation of conditions.
The rise of synthetic data addresses a trifecta of challenges that have long stymied AI development:
The tech community has made significant progress in synthetic data generation, with breakthroughs accelerating in 2023 and 2024. Dario Amodei, CEO of Anthropic, recently emphasized synthetic data’s transformative role in addressing one of AI’s greatest challenges—access to high-quality training data. As the internet becomes saturated with repetitive and AI-generated content, companies like Anthropic are leveraging synthetic datasets to enrich training data and enhance models’ reasoning capabilities.
The power of synthetic data is showcased in a 2024 paper, “ReST Meets ReAct,” which details its application in training large language models for complex, multi-step reasoning tasks. By iteratively generating and refining synthetic datasets, researchers achieved notable improvements in AI agents’ ability to tackle nuanced, compositional questions.
Meanwhile, the open-source CARLA platform continues to push boundaries in synthetic driving environments. Recent updates enable simulations of weather and lighting conditions with unprecedented realism, offering companies the ability to train and evaluate AI models in scenarios that would take decades to observe in the real world.
Despite its advantages, synthetic data is not without its challenges. One major concern is validation: How can we ensure that synthetic datasets accurately reflect the complexities of the real world? If poorly designed, synthetic data can introduce new biases or fail to generalize to real-world scenarios.
Another concern is the growing reliance on synthetic data for training AI models that are themselves used to generate synthetic content. Researchers warn of a potential feedback loop where models trained on synthetic data produce outputs that become less grounded in reality over time, a phenomenon called model collapse.
In fact, a recent study highlights this risk clearly. Researchers found that successive generations of a language model trained on its own synthetic outputs amplified errors, leading to degraded performance. Issues like model collapse raise concerns about the growing reliance on AI-generated data as human-generated content becomes scarce, emphasizing the need for careful curation, diverse datasets, and strategies like watermarking to maintain model integrity and prevent degradation.
The trajectory of synthetic data suggests it will not merely supplement real-world datasets but eventually rival them in importance. As AI models become increasingly capable of generating high-quality, contextually rich synthetic data, we may witness a paradigm shift where synthetic-first approaches dominate fields like natural language processing, computer vision, and robotics.
Consider the potential of diffusion models, a new class of generative AI systems capable of producing photorealistic images and datasets at scale. Tools like OpenAI’s DALL·E 3 and MidJourney’s recent iterations are already blurring the lines between synthetic and real data. Coupled with advancements in homomorphic encryption and privacy-preserving AI, synthetic data could become the default choice for training models in sensitive domains.
Synthetic data is not just a workaround for data scarcity or privacy concerns—it’s a transformative tool reshaping the way AI is developed, trained, and evaluated. From powering safer autonomous vehicles to enabling equitable hiring systems and privacy-compliant tools, its potential is boundless. As the AI field grapples with scaling limitations and ethical challenges, synthetic data stands as one of the most promising solutions on the horizon.
The next decade of AI will not be about data as we know it—it will be about the data we create. How we harness this capability will define the future of artificial intelligence.