Anaconda’s Response to the War in Ukraine
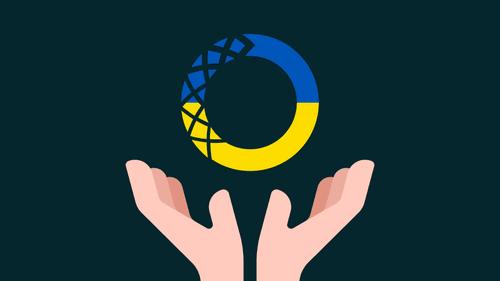
Peter Wang
3min
Over the last decade, open source has fueled many innovations throughout the tech stack. From operating systems to machine learning frameworks, open source has become the de facto choice to leverage the newest and greatest tools. As even staid enterprises turn to open source to power their operations, it’s clear that the benefits aren’t solely found in avoiding vendor lock-in or even cost savings—open source has simply become the superior choice for innovation.
This is because open source is a powerful example of human collaboration, capturing how we can go further together. Labels related to employer, geographical location, and language disappear in the open-source community, and the shared goal of innovation prevails as the guiding force. In the data science field, open source has also enabled ubiquity and access, providing a shared toolkit for practitioners, executives, and students alike to build off of. Open source’s values of democratization and collaboration are intertwined with the data science discipline, and will be key in fueling continued innovation.
The ecology of open source
Open source’s development can be thought of as an ecology, where the vibrancy of the community and its shared mission are what propel the collective forward. For sustainable open source, companies cannot pick the fruits of the community’s labor without tending the overall garden. Instead, companies must recognize the value of investing over time in open source as a community—even when it’s not easy to trace the immediate return on that investment. This way of thinking can seem counterintuitive to traditional “business” logic, but will ultimately pay dividends in the form of accelerated innovation and foundational technical standards.
In a corporate environment, practitioners must be empowered to give back to the community beyond the specific needs of their own company and listen to conversations around how a certain framework can evolve to serve more use cases. A community-based approach to open source will create exponential value in the long run, and the growth of projects such as Dask and Numba illuminate the path for developing common goods.
The open data science movement
It’s no coincidence that many parts of a data scientist’s toolkit have roots in open source. Apache Hadoop’s rise reflected the big data movement, and now many of today’s leading AI applications are powered by TensorFlow. At the core of our work, we rely on notebooks, which are inherently collaborative via link without any necessary installs of software packages. These roots of broad accessibility and open collaboration manifest throughout the best data science work. Just like strong community involvement is critical to the development of innovative open-source software, we believe that the same spirit of collaboration and community is essential to the continued development of data science as a practice.
Because the data science field is relatively young, practitioners have learned to turn to each other to share best practices and standards. In listening to data scientists, we’ve found that online question-and-answer community resources and technical documentation are considered especially helpful for practitioners when they “get stuck.” This is also why industry conferences and virtual communities are a key source of professional development for practitioners of all levels. When new frameworks and methods are being created and discussed at breakneck speed, everyone needs to keep a close eye on the industry’s pulse so they can share what’s worked for them, and learn what could help them. Executives must encourage this culture of continuous learning to not only bring back new trends and ideas to their organizations, but also give back to their communities. For this reason, data science and open source go hand in hand, even beyond the software itself.
Establishing openness as a practice
To formalize the value of community in data science, I believe the industry should create a professional cohort for data scientists. Similar to the American Medical Association or the American Bar Association, a governing body will help data science mature as a unique discipline and epitomize the value of going further together. It will serve to maintain professional standards, uphold best practices, and provide guidance to both employers and educators. The act of coming together and agreeing upon standards will also equip us to better tackle difficult but important issues such as ethics and fairness in data. Such an association will require involvement from all players in the data science industry, regardless of influence or size, to ensure the collective good is being served.
We’re witnessing an incredible rate of innovation in data science right now, bolstered by collaborative work. Innovation atrophies in isolation—or in this case, vendor-defined silos. To keep the magic of open collaboration in data science alive, we must remember that an open-source culture is not the means to an end, but the end that we must strive towards. This will be the only way to ensure that our ceiling of innovation continues to rise.
Talk to one of our experts to find solutions for your AI journey.