2024 State of Data Science and AI
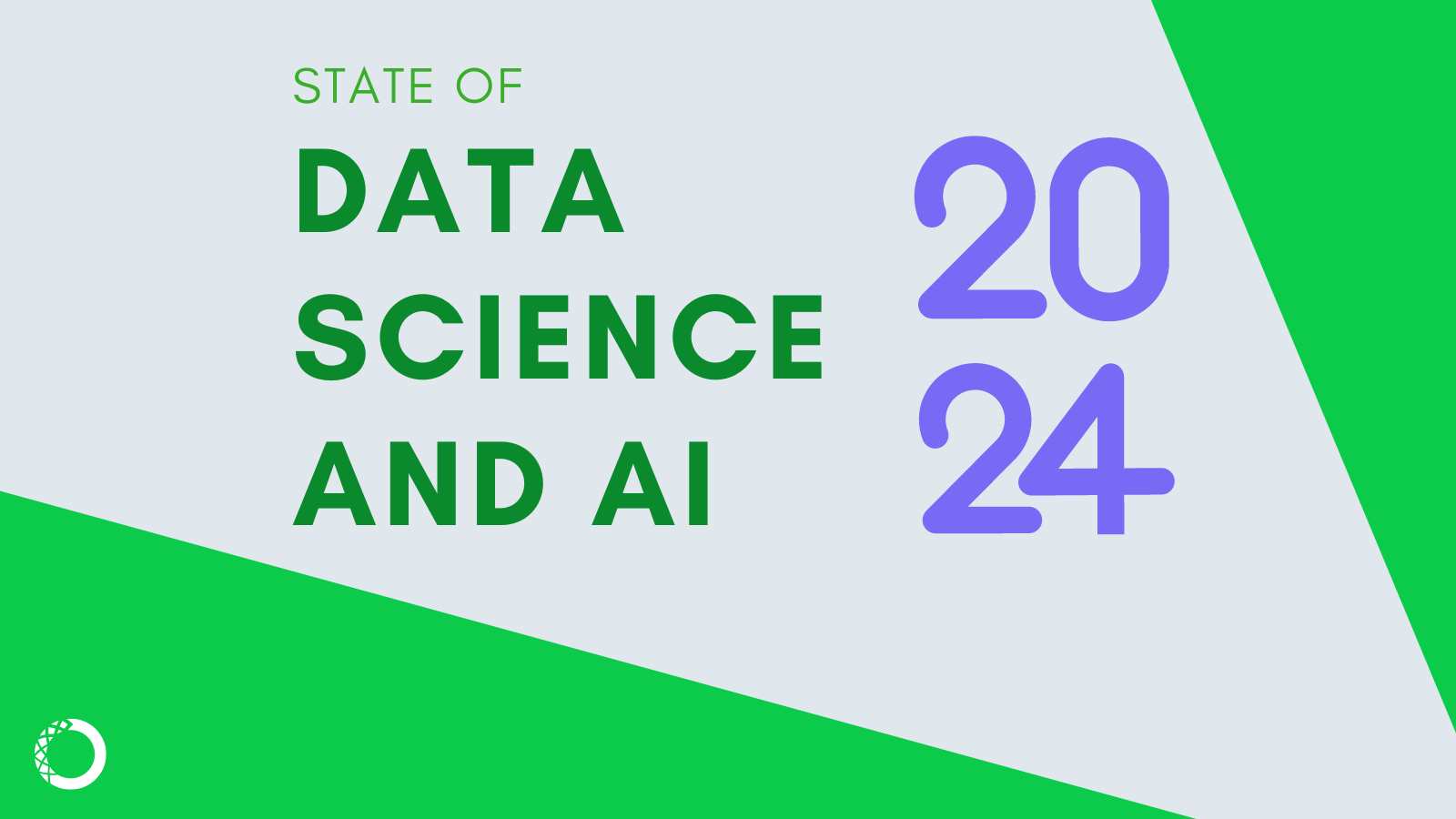
Kodie Dower
Anaconda recently brought together some of the brightest minds in AI for our 2025 AI Predictions Webinar. The panel featured:
● Peter Wang, CAIO of Anaconda
● Greg Jennings, Head of Engineering – AI at Anaconda
● Priyanka Kulkarni, founder and CEO of Casium
● David Pitman, Engineering Leader in AI and Startup and VC Advisor
Together, they explored the trends, innovations, and challenges shaping the future of AI. Let’s dive into some key predictions, takeaways, and insights they shared during the event.
A standout theme of the webinar was the growing momentum behind smaller AI models. These models are set to revolutionize AI applications by enabling on-device processing, which reduces reliance on cloud infrastructure while addressing critical privacy concerns. Panelists noted that larger models might have more features than necessary for most projects, while smaller models could better support more specialized purposes.
“Maybe in 2025, the small models will get so good that we’ll call the larger ones overbuilt models,” said Peter Wang, CAIO of Anaconda. “We’re seeing strong performance coming out of those models, and people are getting better at knowing how to use LLMs. We’re going to see a lot more examples of big models using small models to do very narrow things.”
The panelists emphasized that for task-specific items, starting with the problem and deciding what the model will do to solve it often has better results than relying on a larger model that knows everything on the Internet—which is typically too expansive for a single use case.
Smaller scenarios also lead to easier security and governance compliance, with greater control and more attuned behavior to a given task. And despite what LLMs might pull from, users don’t need a massive, daunting data set to drive results.
“The power of the data you have outshines how much data you have. A good rule of thumb is you could start with 1,000 rows of data specific to what you want to do and see a measurable improvement or change to fine-tune from that,” said David Pitman, Engineering Leader in AI and a Startup and VC Advisor.
The panelists also dispelled the myth that a smaller model can’t handle a complex project. Particularly as companies might start with an LLM before distilling down their use cases, these “distributed hybrid implementations” could utilize on-device image detection or browser extensions that track and summarize everything from a webpage, combining the power of the cloud with more local, on-device AI opportunities.
“I’ve repeatedly heard people start with a large close-sourced model and then build a more fine-tuned model that builds off an open-source model,” said Pitman. “There’s lots of inherent value in using open source around governance, regulatory, compliance, and total cost of ownership. It gives a lot more flexibility than a closed-source model.”
“You don’t need it to be great. You need it to be great at the specific things you want it to do,” added Greg Jennings, Head of Engineering – AI at Anaconda.
Another major trend discussed was the increasing investment in multimodal models. These sophisticated AI systems integrate data from multiple sources, including text, images, audio, and video, pushing the boundaries of AI capabilities.
“Multimodal models are here, and they’re just going to grow in 2025,” said Priyanka Kulkarni, founder and CEO of Casium. “In the case of legal or immigration, your data is images, your passport picture, your healthcare records. We’re dealing with multilingual and multimodal. People submit audio clips to show the government they’re experts in an area. We’ve seen similar workflows with startups in healthcare and retail. A lot of these elements with multimodality are already being experimented with, and we’re seeing a lot more happening with vision, as well. Next year is going to be multimodal on steroids.”
Pitman agreed that image, video, and multimodal models are the most exciting but said there’s still a mountain to climb before they reach an enterprise-ready state. He encouraged attendees to consider modality beyond the obvious.
“People are overlooking other forms of modality, like time series data and other types of structured data models,” he said. “It bridges that gap between text and structured data, and it’s easier to act and work upon that.”
“Multimodality is beyond text to text,” Wang added. “It’s really driving the change in the zeitgeist. This is AI breaking out into the world and being a part of embedded intelligence we can bring with us.”
The panel also touched on groundbreaking advancements in “world” models, which create complex simulations to help AI agents make more intelligent decisions. Jennings referenced a talk he heard at this year’s NeurIPS conference that said, “The era of pre-training is over” because we only have one Internet for models to pull from. But there’s another area that Jennings thinks we should spend time on that can complement these world models.
“What’s relatively unexplored is a synthetic data set to generate additional data sets,” he said. “As these models continue to get better, their ability to do a better job of generating image, video, and audio content more in line with the theme and input given to them can actually help reinforce, build, and grow AI systems overall.”
The panelists also talked about showcasing the value of AI. With so many different technologies, products, and models, it can be easy to get stuck in the mud in terms of focusing on the ROI of an investment or project.
“You’re not going to help fix everything. If there’s a specific scenario, that’s where AI can come and step in. Being results-focused is going to be the key metric,” Kulkarni said.
Wang also noted the ever-evolving landscape. He stressed the importance of robust validation, with companies continuing to check in on what they’ve built and ensuring it’s still performing better than something else. Looking at the trade-off between complexity and performance is a helpful mindset.
“Based on what we’ve seen in the last one to one-and-a-half years, a way of doing things that works great now may be completely obviated in the future,” Wang said. “The whole technological colossus of all of this happening is to help people have better impact at greater efficiency and lower cost.”
Wang emphasized many company leaders fall for the “trap” of measuring the efficacy of a technology, but creating KPIs around how it helps you achieve business outcomes is a superior approach. Kulkarni aligned with that mindset, saying she and her team at Casium measure success based on how many immigrants they can help obtain visas.
“Smart people should be able to work on their dreams real quick,” she said. “We’re able to help people get business visas in days because we don’t work like the incumbents. We’ve transitioned to something more outcome-focused.”
The webinar concluded with an interactive Q&A session, with attendees asking questions to help drive their business goals. The panelists provided actionable insights, reinforcing practical strategies and opportunities for businesses in 2025.
“One of the best things about AI is so much of it has happened in the open,” said Pitman. He called out a few resources, including innovation competitions in Kaggle and learning series from sources like fast.ai. He stressed one of the best ways to expand on AI is to start experimenting and playing around with it.
“Everybody I know who’s at the forefront of AI is constantly relearning everything happening every three months,” he said. “We’re always trying to sharpen our skills. You don’t just accumulate this knowledge once and get to coast on it for years and years. There are a lot of people talking about stuff to learn.”
Anaconda also has its own Anaconda Learning library, with many free resources to help users get started on their AI journeys.
Agentic AI is the simple idea that we can take LLMs and make them into modular units that can be composed into a workflow, something that a human can oversee. These AI agents can help with the “boring” AI tasks like automating document processing, streamlining data cleaning, and delivering precise results for specific projects. Wang highlighted his two-pronged approach in this space.
“First, I look at people documenting quite specifically about their successes. I look for those people who have built an end-to-end working thing close to what I’m trying to accomplish,” Wang said. Then, I look at the simplest possible thing I could build that would work end-to-end. Until you build something simple yourself, you’re going to drown and spin your wheels in the mud of frameworks.”
Pitman, who’s launched seven different companies, added the three things he keeps in mind for a successful agentic AI approach:
Focusing on these considerations can improve agentic AI strategies in 2025 and beyond.
Kulkarni acknowledged that there have been some impressive strides in artificial general intelligence (AGI), but there’s still some time to go until it reaches its peak. She estimated it would take about 15 years—right around 2040—for that peak to hit, and Pitman and Jennings agreed.
“We’re getting really good at neuro tasks, reasoning, generalizing in some models, but not all of them,” she said. “General intelligence is across domains, modalities, and different tasks. Data and compute will play interesting roles, and it’s also about safety and ethics. We haven’t thought about what it’s like to have systems in this world and the way they work.”
Jennings also called out the moving target around AGI. “The models we have now aren’t specifically trained on cause and effect, and we have to incorporate that back to achieve true AGI,” he said, noting the multimodal nature emerging in the space could help close the gap by bringing more of that data in.
If you missed the live session, don’t worry! A recording of the webinar is available here. Stay tuned for more thought leadership and insights from Anaconda as we continue to explore the evolving world of AI.