A Comprehensive List of Python in Excel Resources
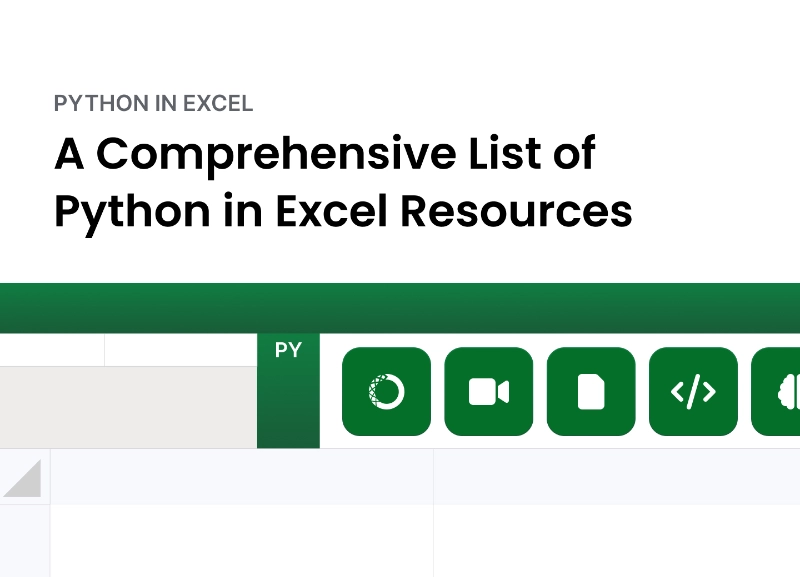
There are no solved problems; there are only problems that are not yet solved.” – [Attributed to, possibly apocryphally] John Carmack
At Anaconda, we’re constantly exploring how to help developers get the most from AI tools. When generating code with Large Language Models (LLMs), the quality of output can vary dramatically based on subtle prompt differences. But what if there’s a more systematic approach to optimizing these interactions?
Intrigued by recent reports that found LLMs capable of reasoning and overperforming when assigned specific roles, we set out to answer a simple yet powerful question: Can we influence not just what code LLMs generate, but how they generate it, by invoking the mindsets of legendary programmers?
Using Anaconda’s Assistant-enabled notebooks, we set out to explore whether the latent space of LLMs contains distinct “programming personalities” that could be channeled for specific coding tasks. The results weren’t just surprising—they fundamentally challenge how we might approach AI-assisted development going forward.
My journey began with two distinct but equally compelling sources of inspiration.
First, Max Woolf’s insightful blog post, “Can LLMs write better code if you keep asking them to “write better code”?” caught my attention. Woolf’s experiment elegantly demonstrated that even a vague, iterative prompt like “make it better” could coax an LLM into producing progressively more optimized code. He started with a basic Python solution to a numerical problem and, through repeated prompting, ended up with a highly optimized, multi-threaded, and even “enterprise-ready” implementation, complete with logging and graceful shutdown.
This highlighted two key points. First, LLMs possess optimization capabilities that go beyond simple code generation. They can, to some extent, “reason” about code efficiency, even without explicit instructions or a profiler. Second, while iterative prompting can improve code, it can also lead to unintended consequences, like over-engineering or adding unnecessary features. Basically, just asking an LLM to ‘make it better’ is like giving a vague instruction to a genie – you might get what you wish for, but not in the way you expect.
The inspiration came from seeing the o1 jailbreak system prompt: the so-called “Carmack prompt”. This prompt, designed to elicit a John Carmack-like response from an LLM as it reasoned, asked the model to pursue:
The “Carmack prompt” wasn’t just about writing correct code; it was about writing optimal code – the kind John Carmack is famous for since Doom.
These observations led to a compelling hypothesis: Could we influence the quality and style of generated code by asking LLMs to channel specific programming legends?
To test this hypothesis, I needed a well-defined problem with clear performance metrics.
Over COVID, years ago, I created a Wordle solver. Originally, this was so I would have a chance to compete with my sister in law in Ireland at the Irish language Wordle game. Now, I don’t know Irish, but I do know how to write a solver for it given several thousand possible words!
A Wordle solver needs to:
This task is complex enough to allow for significant variations in algorithmic approach and optimization, yet simple enough to evaluate effectively..
The experiment was conducted using the Anthropic Claude 3.5 Sonnet model (claude-3-5-sonnet-20241022), accessed through the AWS Bedrock API—the same model that backs our Anaconda Assistant.
I chose Claude 3.5 Sonnet for its exceptional prompt adherence, a crucial characteristic when attempting to guide the model’s behavior in such specific ways. As Max Woolf and others have noted, Claude 3.5 Sonnet consistently excels in following instructions, making it an ideal candidate for this experiment.
The API calls were configured with the following parameters:
To ensure a fair comparison, each “personality” was tasked with implementing the same WordleSolver class in Python, with almost exactly the same prompt. This class had a strictly defined interface:
class WordleSolver:
def __init__(self, word_list: list[str]):
"""
Initializes the solver with a list of possible words.
"""
pass
def make_guess(self) -> str:
"""
Returns the solver's next guess.
"""
pass
def submit_guess_and_get_clues(self, clues: list[str], guess: str):
"""
Updates the solver's internal state based on clues.
"""
pass
def reset(self):
"""
Resets the solver's internal state.
"""
pass
Crucially, there was no evaluate_guess method. Clue generation was handled externally by a separate WordleSimulator class. This forced the solvers to focus solely on their internal strategy for selecting guesses and filtering the word list based on the provided feedback. They couldn’t “cheat” by replicating the game’s logic.
I compared a range of personalities, each representing a distinct approach to programming:
The prompt for each personality was almost identical, differing only in the introductory phrase. For example:
The rest of the prompt detailed the strict interface requirements, the prohibition of an evaluate_guess method, and the need for a single, well-formatted code block output. This was to help ensure differences in generated code are attributed to our channeled personalities.
Each solver was evaluated against a fixed list of 500 English words, running 500 simulated Wordle games. I measured:
These metrics provided a quantitative assessment of each solver’s effectiveness and efficiency. The results were both surprising and insightful.
Each solver was evaluated against a fixed list of 500 English words, running 500 simulated Wordle games, measuring win rate, average attempts, and average guess time.
The table below summarizes the key findings:
Personality | Win Rate (%) | Avg Attempts | Avg Guess Time (ms) | Key Observations |
---|---|---|---|---|
No Person 1 | 0.0 | inf | 2.510 | Failed completely. Highlights the importance of even basic strategy. Overly simplistic, with critical logical flaws. |
No Person 2 | 63.0 | 4.89 | 2.823 | Basic strategy, but slow due to inefficient clue checking. Relied heavily on list iteration. |
No Person 3 | 84.6 | 4.26 | 4.169 | Improved strategy, using letter frequency. Still relatively slow, demonstrating diminishing returns without personality guidance. |
No Person 4 | 85.8 | 4.25 | 1.959 | Surprisingly good performance. Shows Claude 3.5 Sonnet’s inherent capabilities. Used both letter and position frequencies. |
Carmack | 85.0 | 4.36 | 2.066 | Very fast and efficient. Used sets for O(1) lookups, precomputed letter frequencies, concise code. Prioritized raw performance. |
Knuth | 82.8 | 4.27 | 2.507 | Well-documented, clear code. Prioritized correctness and readability. Used a scoring system based on letter/position frequencies. |
Johnson | 86.8 | 4.32 | 1.690 | Fastest solver overall. Focused on precise constraints and efficient filtering. Used sets for letter constraints, elegant logic. |
Fowler | 48.6 | 4.92 | 2.586 | Emphasized readability, maintainability. Performance suffered significantly. Highlights trade-off between clean code and speed. |
Linus | 48.2 | 4.92 | 1.424 | Reasonably fast, but the win rate was relatively low. |
Torvalds | 0.0 | inf | 5.112 | Failed completely. Overly simplistic frequency analysis. Slow, but correct, clue checks. |
Guido | 61.8 | 4.87 | 4.304 | Slower, and less accurate than expected. |
Hybrid | 85.4 | 4.26 | 2.593 | Combined strengths of Carmack, Knuth, and Johnson. Good balance of speed and correctness. Blended different optimization techniques. |
The differences between the solvers weren’t just about numbers; they were also about how the code was written. Here’s a closer look at the stylistic variations:
One of the most illuminating aspects of the experiment was seeing how different personas tackled the same sub-problem within the Wordle solver. Let’s focus on the core challenge: filtering the list of possible words based on the clues (green, yellow, gray) received after a guess. This is where the algorithmic and stylistic differences become most apparent.
We’ll compare three key approaches:
Sub-Problem: Filtering Possible Words
The submit_guess_and_get_clues method is responsible for this filtering. It receives the clues (a list of “Green”, “Yellow”, “Gray” strings) and the guess (the word that was guessed). It must update the possible_words list, removing any words that are now inconsistent with the clues.
1. Katherine Johnson: Constraint-Based Filtering
Here’s a simplified version of Katherine Johnson’s approach (from the WordleSolverKatherine class):
def submit_guess_and_get_clues(self, clues: list[str], guess: str):
must_contain = []
must_not_contain = set()
position_constraints = [set() for _ in range(5)]
exact_positions = {}
for i, (clue, letter) in enumerate(zip(clues, guess)):
if clue == "Green":
exact_positions[i] = letter
elif clue == "Yellow":
must_contain.append(letter)
position_constraints[i].add(letter)
else: # Gray
if (letter not in must_contain and
letter not in exact_positions.values()):
must_not_contain.add(letter)
new_possible_words = []
for word in self.possible_words:
valid = True
# ... (checks for exact_positions, position_constraints,
# must_contain, must_not_contain) ...
if valid:
new_possible_words.append(word)
self.possible_words = new_possible_words
Key Features:
2. John Carmack: Optimization-Focused Filtering
Here’s a simplified version of John Carmack’s approach (from the WordleSolverCarmack class):
def submit_guess_and_get_clues(self, clues: list[str], guess: str):
green_positions = {i for i, clue in enumerate(clues) if clue == "Green"}
yellow_positions = {i for i, clue in enumerate(clues) if clue == "Yellow"}
gray_letters = {guess[i] for i, clue in enumerate(clues)
if clue == "Gray" and guess[i] not in
{guess[j] for j in green_positions | yellow_positions}}
self.possible_words = {
word for word in self.possible_words
if all(word[i] == guess[i] for i in green_positions) and
all(word[i] != guess[i] and guess[i] in word
for i in yellow_positions) and
not any(letter in gray_letters for letter in word)
}
Key Features:
3. Donald Knuth: Clarity and Documentation
Here’s a simplified version of Donald Knuth’s approach (from the WordleSolverKnuth class):
def submit_guess_and_get_clues(self, clues: list[str], guess: str):
new_possible = []
for word in self.possible_words:
if self._is_word_consistent(word, guess, clues):
new_possible.append(word)
self.possible_words = new_possible
def _is_word_consistent(self, word: str, guess: str, clues: list[str]) -> bool:
# ... (detailed logic with comments explaining each step) ...
return True
#Inside the Knuth _is_word_consistent
# Check green clues first
for i, (clue, guess_letter) in enumerate(zip(clues, guess)):
if clue == "Green" and word[i] != guess_letter:
return False
# Track yellow letters
remaining_letters = list(word)
# Remove green positions
for i, (clue, guess_letter) in enumerate(zip(clues, guess)):
if clue == "Green" and guess_letter in remaining_letters:
remaining_letters.remove(guess_letter)
# Check yellow clues
for i, (clue, guess_letter) in enumerate(zip(clues, guess)):
if clue == "Yellow":
if word[i] == guess_letter: # Yellow letter can't be in same position
return False
if guess_letter not in remaining_letters: # Yellow letter must be somewhere
return False
remaining_letters.remove(guess_letter)
# Check gray clues
for i, (clue, guess_letter) in enumerate(zip(clues, guess)):
if clue == "Gray" and guess_letter in remaining_letters:
return False
return True
Key Features:
These findings have significant implications for anyone working with AI in a development context:
The code each personality generates tells a unique story. Knuth’s reads like a well-documented mathematical proof. Carmack’s is a Ferrari of a program: sleek, fast, and demanding expertise to maintain. But Katherine Johnson’s combines mathematical elegance with ruthless pragmatism, creating a web of constraints that efficiently filters invalid options.
The implications extend far beyond our Wordle experiment. We’ve discovered something profound in the latent space of these models—a kind of crystallized essence of human programming wisdom, ready to be applied to new problems.
This experiment suggests a powerful new approach to prompt engineering for Anaconda users working with Python and AI tools. Instead of focusing solely on what you want the AI to do, consider who you want the AI to channel while doing it. The right persona can make the difference between mediocre and exceptional code generation.
For those interested in further exploring how different AI models perform on specific tasks, our recent post on evaluating specialized SQL models provides additional insights into the capabilities and limitations of various LLMs. These evaluation methodologies can help you determine which models might work best for your particular needs.
As we continue exploring the intersection of AI and software development at Anaconda, we’re excited to integrate these findings into our tools and workflows. The latent space holds more secrets waiting to be discovered—and with the right prompting strategies, we can unlock them together.
Try applying different personas in your own AI coding experiments using the Anaconda Assistant, an AI Python coding companion, and AI Navigator, our gateway to more than 200 pre-trained LLMs. Share your findings with us on GitHub or our community forums. For enterprise users, our Professional Services team can help develop custom prompt engineering strategies tailored to your development needs.